How AI solution’s maintenance is different from traditional software
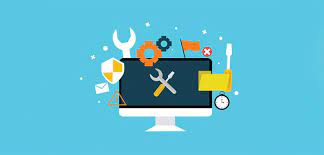
IDC predicts that up to 88 percent of all AI and ML projects will fail during the test phase[1]. A major reason is that AI solutions are difficult to maintain. In this post, I will highlight how the maintenance of AI solutions is different and why MLOps is important.
Some business executives and even engineers think that when an AI solution is deployed, you’re done. But most of the time you may only be halfway to the goal. Substantial work lies ahead in monitoring, maintaining, and optimizing the system. I will list down some of the maintenance challenges below:
Data drift:
Data drift is one of the top reasons model accuracy degrades over time. Data drift is the change in model input data that leads to model performance degradation. The model was trained on a certain distribution of data, but this distribution changes over time. Causes of data drift include:
- Upstream process changes, such as customer profile and behavioral data model is updated that changes the incoming data into AI model for customer segmentation or personalized marketing
- Data quality issues, such as a broken sensor always reading 0 or data from some form getting corrupted because of some bug that will send bad data into the model
- Natural drift in the data, such as pre-covid vs post-covid changes in user behavior
For example, a model may have learned to estimate demand for a mass transit system from historical pre-covid data, but covid caused unprecedented changes to riders patterns, so the model’s accuracy will degrade. AI model will need to be retrained to reflect new realities.
Concept drift.
Concept drift is a major consideration for ensuring the long-term accuracy of AI solutions. Concept drift can be understood as changes in the relationship between the input and target output. The properties of the target variables may evolve and change over time. The model was trained to learn an x->y mapping, but the statistical relationship between x and y changes, so the same input x now demands a different prediction y.
Concept drift can be also be caused by the redefinition of the y variable. For instance, a model detects construction workers who wander into a dangerous area without a hard hat for more than 5 seconds. But safety requirements change, and now it must flag hatless workers who enter the area for more than 3 seconds. This will require retraining of the model.
Traditional software engineering maintenance:
It's not a major challenge, but I wanted to include this for the sake of completeness. Data plumbing, interfaces, and infrastructure of the AI solutions are similar to traditional software engineering products, So traditional maintenance will be needed for AI solutions as well, such as:
- Infrastructure: Cloud or on-prem infrastructure maintenance and monitoring
- Updates: Libraries and OS updates
- AI Solution Interface maintenance: AI solutions always need to have an interface through which the end-users can interact with it. It could be a chatbot, BI dashboard, web application or mobile app. Maintenance of these components is necessary as interfaces evolve with time based on new business requirements
Importance of MLOps
Detecting concept and data drift is challenging, because AI systems have unclear boundary conditions. For traditional software, boundary conditions — the range of valid inputs — are usually easy to specify. But for AI software trained on given data distribution, it’s challenging to recognize when the data distribution has changed sufficiently to compromise performance.
This problem is exacerbated when one AI system’s output is used as another AI’s input in what’s known as a multi-step AI solution. For example, one system may detect people and a second may determine whether each person detected is wearing a hard hat. If the first system changes — say, you upgrade to a better person detector — the second may experience data drift, causing the whole system to degrade.
Over the past few decades, software engineers have developed relatively sophisticated tools for versioning, maintaining, and collaborating on code. We have processes and tools that can help you fix a bug in code that a teammate wrote 2 years ago. But AI systems require both maintenances of code and data.
Ask yourself:
- Is the AI model’s health being monitored in real-time?
- Can the model be trained automatically, once the performance has been degraded to a certain threshold?
- Is training datasets and models being versioned?
- Can you monitor and detect data drift?
If the answer to any of the above questions is no, know that your AI solution will decay our time and will be costly to maintain.
For easy to maintain AI solutions, MlOps are necessary. MLOps helps drive business value by fast-tracking the experimentation process and development pipeline, improving the quality of model production — and making it easier to monitor and maintain production models and manage regulatory requirements.