How to do data and ROI feasibility of a machine learning based forecasting solution
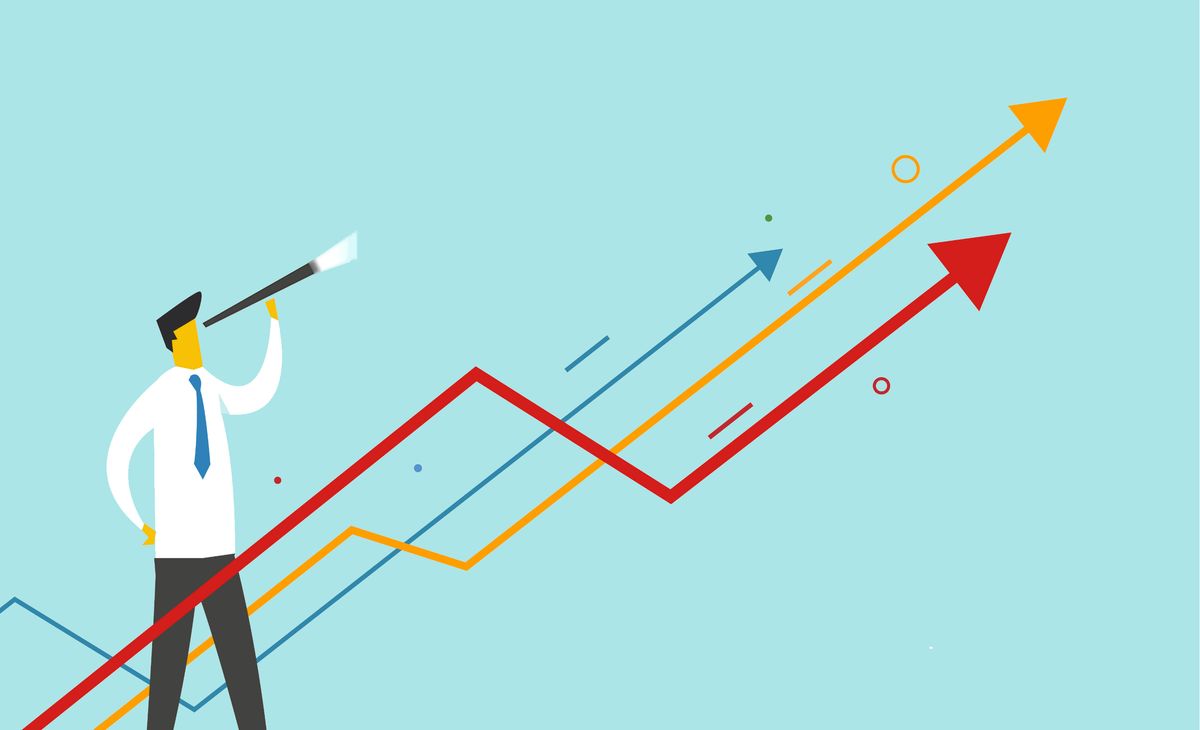
A list of key questions that will help asses business and technical feasibility of a machine learning solution
Lets take an example of a machine learning and deep learning based forecasting solution. Imagine that you are the Machine learning engineer and want to asses a forecasting use case from two perspectives
- Expected business ROI that the solution will generate, It ideally should be a dollar value.
- Technical feasibility like is the right data available or is acceptance criteria is achievable with the given data set
Forecasting is the science of predicting the future. Accurate forecasting is relevant in a broad set of business scenarios, such as:
- Product demand
- Cash flow
- Inventory planning
- Staffing at call centers
- The selling price of crops
- Deliveries per zip code etc.
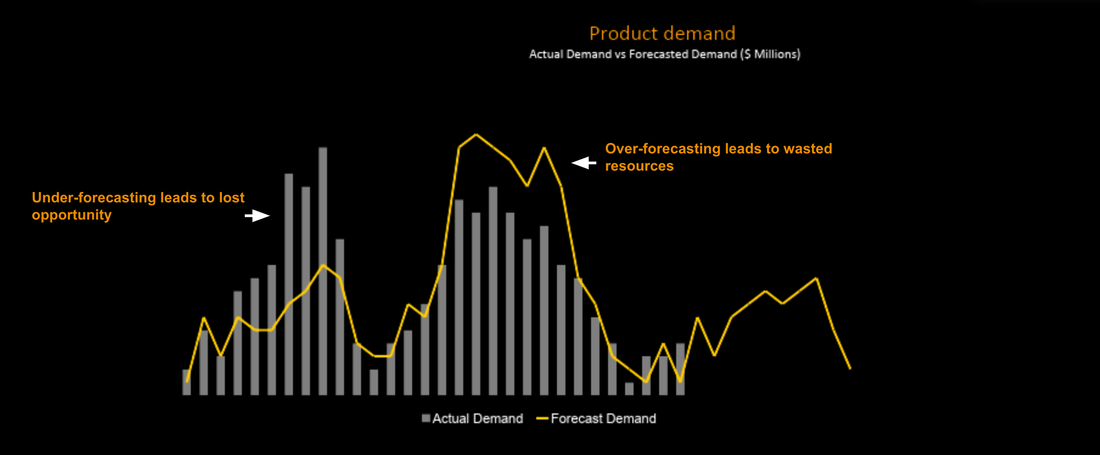
A sample of business challenges specific to transportation needs, accurate forecasting can solve:
- How many on-demand rental bikes are needed at a time around a 3 block radius?
- How many on the ground operation specialists are needed to transfer bikes from one location to the other?
- How many trucks are needed daily to deliver goods on time to a warehouse in a specific
Discovery Questions
Here are a set of discovery questions that should be used for the technical and business feasibility of the use case:
- What tools do you use to generate the forecasts today?[look for excel that will imply that there is an opportunity with significant improvement]
- How do you generate these forecasts today? explain the process? [look for download data, run in Excel thru a static algorithm, add a buffer of (say) +/-20%, this implies that just automating the process significant savings in manual labor can be achieved]
- Please share the sample inputs and outputs for forecasting? [This will help you to look at the granuality]
- How accurate are the predictions?[Most of the time business team will not be measuring the accuracy]
- What are the areas of improvement in the current process, that you have in mind?[It can be accuracy or automation of the process of both]
- What data sets are you using currently for the forecast? What additional data sets would you like to incorporate?
- How much would it benefit you to produce a forecast that is, say, 20% more accurate?[This will help clarify the business impact for the investment they are going to make]
Feel free to ask questions in the comments section.